
- #Nvidia cuda toolkit how to
- #Nvidia cuda toolkit serial
- #Nvidia cuda toolkit update
- #Nvidia cuda toolkit software
ĬUDA supports a single and latest KylinOS release version. ĬUDA supports a single and latest Fedora release version. Please refer to the support lifecycle for these OSes to know their support timelines.ĬUDA supports a single and latest Debian release version. ĬUDA support for Ubuntu 18.04.x, Ubuntu 20.04.x, Ubuntu 22.04.x, RHEL 7.x, RHEL 8.x, RHEL 9.x, CentOS 7.x, Rocky Linux 8.x, Rocky Linux 9.x, SUSE SLES 15.x and OpenSUSE Leap 15.x will be until the standard EOSS as defined for each OS. L4T provides a Linux kernel and a sample root filesystem derived from Ubuntu 20.04. Minor versions of the following compilers listed: of GCC, ICC, NVHPC, and XLC, as host compilers for nvcc are supported. For platforms that ship a compiler version older than GCC 6 by default, linking to static cuBLAS and cuDNN using the default compiler is not supported. Newer GCC toolchains are available with the Red Hat Developer Toolset. On distributions such as RHEL 7 or CentOS 7 that may use an older GCC toolchain by default, it is recommended to use a newer GCC toolchain with CUDA 11.0. Note that starting with CUDA 11.0, the minimum recommended GCC compiler is at least GCC 6 due to C++11 requirements in CUDA libraries, such as cuFFT and CUB. įor Ubuntu LTS on x86-64, the Server LTS kernel (for example, 4.15.x for 18.04) is supported in CUDA 12.0. Ī list of kernel versions including the release dates for SUSE Linux Enterprise Server (SLES) is available at. The following notes apply to the kernel versions supported by CUDA:įor specific kernel versions supported on Red Hat Enterprise Linux (RHEL), visit.
#Nvidia cuda toolkit update
Native Linux Distribution Support in CUDA 12.1 Update 1 Please review the footnotes associated with the table. The following table lists the supported Linux distributions. The CUDA development environment relies on tight integration with the host development environment, including the host compiler and C runtime libraries, and is therefore only supported on distribution versions that have been qualified for this CUDA Toolkit release. To use NVIDIA CUDA on your system, you will need the following installed:Ī supported version of Linux with a gcc compiler and toolchain
#Nvidia cuda toolkit how to
This guide will show you how to install and check the correct operation of the CUDA development tools. The on-chip shared memory allows parallel tasks running on these cores to share data without sending it over the system memory bus. These cores have shared resources including a register file and a shared memory. This configuration also allows simultaneous computation on the CPU and GPU without contention for memory resources.ĬUDA-capable GPUs have hundreds of cores that can collectively run thousands of computing threads. The CPU and GPU are treated as separate devices that have their own memory spaces. As such, CUDA can be incrementally applied to existing applications.
#Nvidia cuda toolkit serial
Serial portions of applications are run on the CPU, and parallel portions are offloaded to the GPU. Support heterogeneous computation where applications use both the CPU and GPU. With CUDA C/C++, programmers can focus on the task of parallelization of the algorithms rather than spending time on their implementation. Provide a small set of extensions to standard programming languages, like C, that enable a straightforward implementation of parallel algorithms. It enables dramatic increases in computing performance by harnessing the power of the graphics processing unit (GPU).ĬUDA was developed with several design goals in mind: Introduction ĬUDA ® is a parallel computing platform and programming model invented by NVIDIA ®.
#Nvidia cuda toolkit software
These containers can be used for validating the software configuration of GPUs in the system or simply to run some example workloads.The installation instructions for the CUDA Toolkit on Linux.
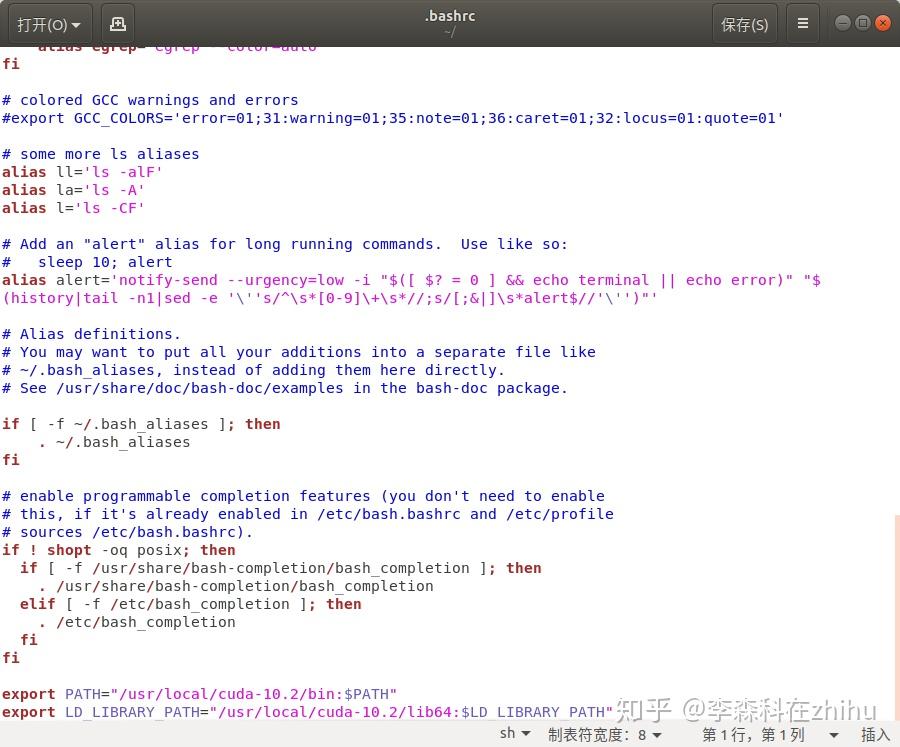
The collection includes containerized CUDA samples for example, vectorAdd (to demonstrate vector addition), nbody (or gravitational n-body simulation) and other examples. CUDA Samples : This is a collection of containers to run CUDA workloads on the GPUs.The CUDA container images provide an easy-to-use distribution for CUDA supported platforms and architectures. The CUDA Toolkit includes GPU-accelerated libraries, a compiler, development tools and the CUDA runtime. CUDA Containers : The CUDA Toolkit from NVIDIA provides everything you need to develop GPU-accelerated applications.The toolkit includes GPU-accelerated libraries, debugging and optimization tools, a C/C++ compiler, and a runtime library to deploy your application. With the CUDA Toolkit, you can develop, optimize, and deploy your applications on GPU-accelerated embedded systems, desktop workstations, enterprise data centers, cloud-based platforms and HPC supercomputers. The CUDA Toolkit provides the core, foundational development environment for creating high performance NVIDIA GPU-accelerated applications.
